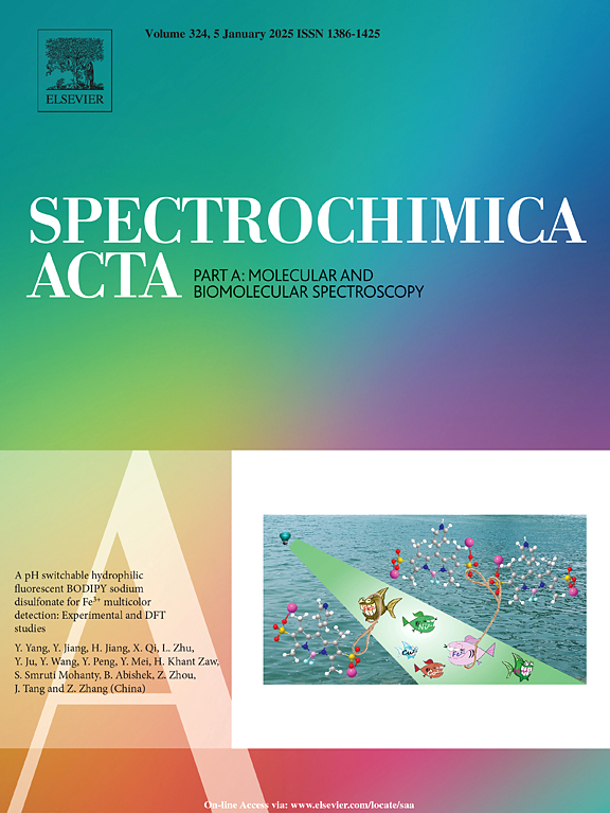
Published at: Spectrochimica Acta Part A: Molecular and Biomolecular Spectroscopy
Abstract
Abstract Among the most prevalent and detrimental bacteria causing urinary tract infections (UTIs) is Klebsiella (K.) pneumoniae. A rapid determination of its antibiotic susceptibility can enhance patient treatment and mitigate the spread of resistant strains. In this study, we assessed the viability of using infrared spectroscopy-based machine learning as a rapid and precise approach for detecting K.
pneumoniae bacteria and determining its susceptibility to various antibiotics directly from a patient's urine sample. In this study, 2333 bacterial samples, including 636 K.
pneumoniae were investigated using infrared micro-spectroscopy. The obtained spectra (27996spectra) were analyzed with XGBoost classifier, achieving a success rate exceeding 95 % for identifying K. pneumoniae.
Moreover, this method allows for the simultaneous determination of K. pneumoniae susceptibility to various antibiotics with sensitivities ranging between 74 % and 81 % within approximately 40 min after receiving the patient's urine sample.
Infrared spectroscopy-based machine learning algorithms
Share a link using:
https://www.afeka.ac.il/en/industry-relations/research-authority/infrared-spectroscopy-based-machine-learning-algorithms/WhatsApp
Facebook
Twitter
Email
https://www.afeka.ac.il/en/industry-relations/research-authority/infrared-spectroscopy-based-machine-learning-algorithms/