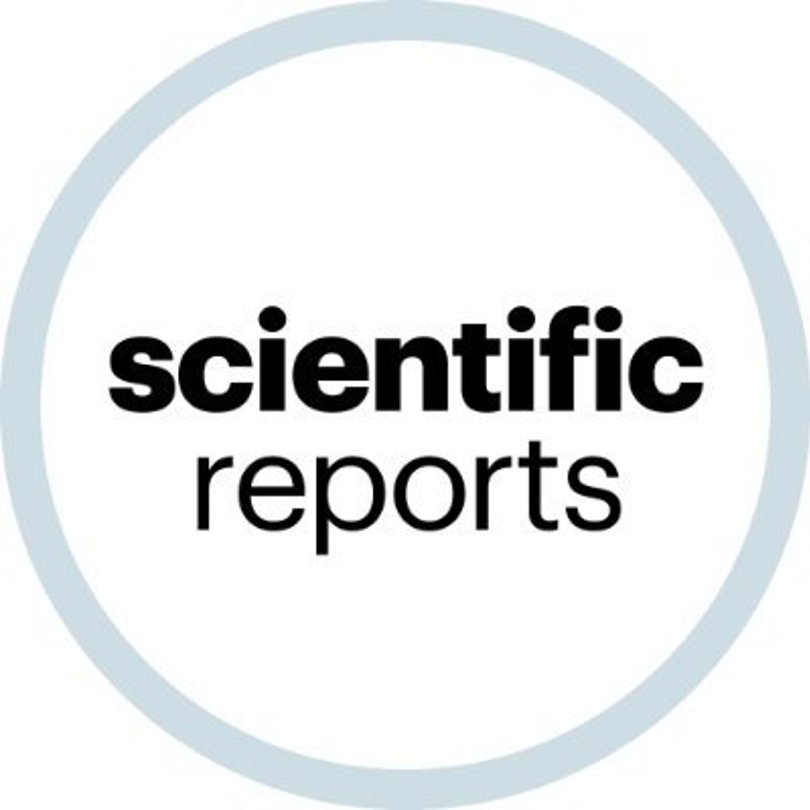
Authors From Afeka: Dr. Alex Segal
Abstract
Prosthetic devices are vital for enhancing personal autonomy and the quality of life for amputees. However, the rejection rate for electric upper-limb prostheses remains high at around 30%, often due to issues like functionality, control, reliability, and cost. Thus, developing reliable, robust, and cost-effective human-machine interfaces is crucial for user acceptance. Machine learning algorithms using Surface Electromyography (sEMG) signal classification hold promise for natural prosthetic control. This study aims to enhance hand and wrist movement classification using sEMG signals, treated as time series data. A novel approach is employed, combining a variation of the Random Convolutional Kernel Transform (ROCKET) for feature extraction with a cross-validation ridge classifier. Traditionally, achieving high accuracy in time series classification required complex, computationally intensive methods. However, recent advances show that simple linear classifiers combined with ROCKET can achieve state-of-the-art accuracy with reduced computational complexity. The algorithm was tested on the UCI sEMG hand movement dataset, as well as on the Ninapro DB5 and DB7 datasets. We demonstrate how the proposed approach delivers high discrimination accuracy with minimal parameter tuning requirements, offering a promising solution to improve prosthetic control and user satisfaction.
Classification of hand and wrist movements via surface electromyogram using the random convolutional kernels transform
Share a link using:
https://www.afeka.ac.il/en/industry-relations/research-authority/classification-of-hand-and-wrist-movements-via-surface-electromyogram-using-the-random-convolutional-kernels-transform/WhatsApp
Facebook
Twitter
Email
https://www.afeka.ac.il/en/industry-relations/research-authority/classification-of-hand-and-wrist-movements-via-surface-electromyogram-using-the-random-convolutional-kernels-transform/